Explainable AI
"Explainable AI" (xAi) or "explainability" is when you design and build systems that can explain their decisions. Turns out I do this right now.
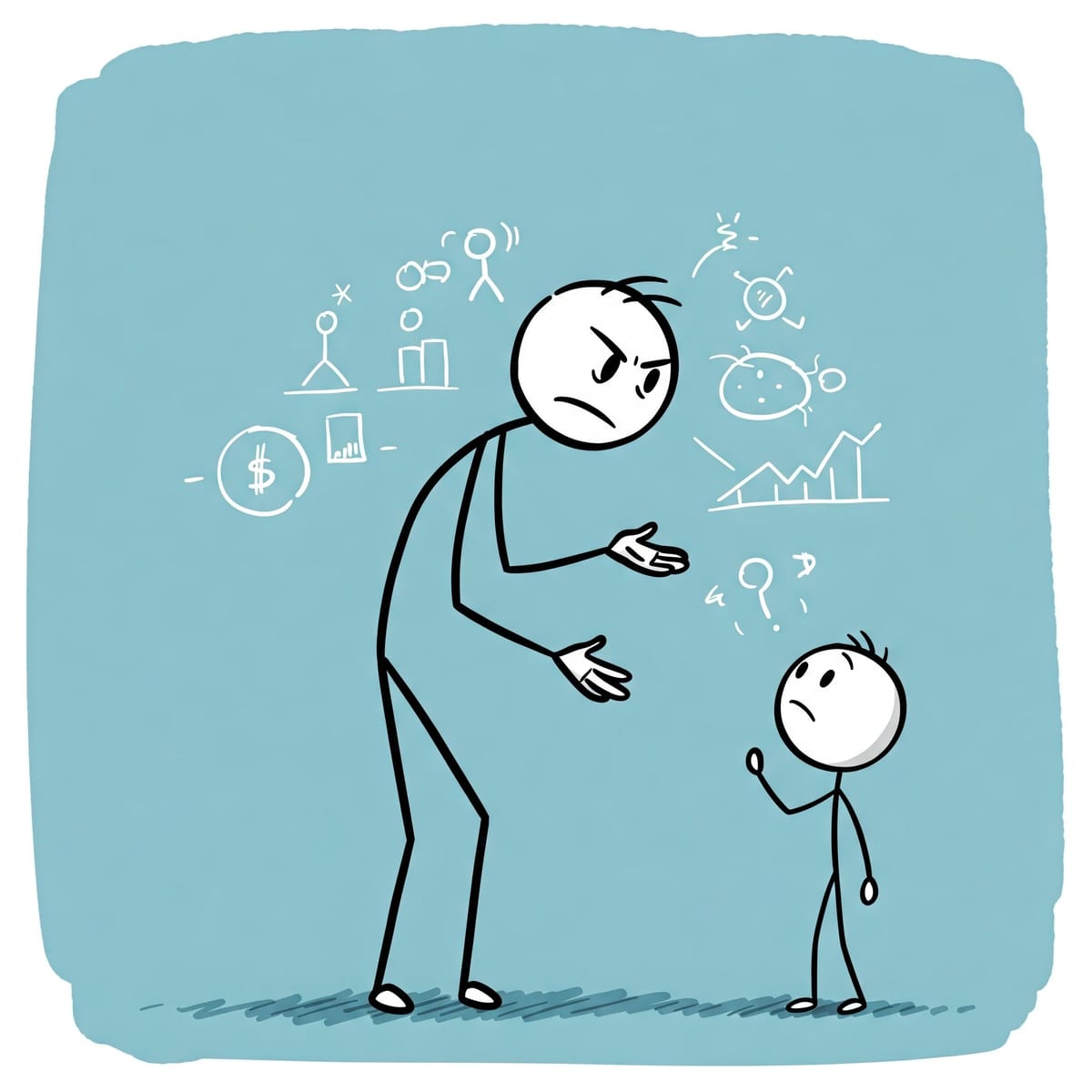
At the very end of Thoughts on The Worlds I See, I mention a new field of research called Explainable AI (xAi) or Explainability.
The idea is simple enough:
At STELLA Automotive AI, we handle phone conversations (also SMS + Web conversations) between customers and car dealerships. STELLA answers the phone, interacts with the customer, and can help them book a service appointment. This interaction involves transcribing what the customer says, understanding the customer's intent, asking follow up questions and then directing them somewhere.
Many times per day dealers ask why a particular call outcome happened. “Why did STELLA send the caller to finance when they asked for service?” They want us to explain the choices STELLA made. They want to understand if something is off (either in our system or in theirs).
How to “explain” choices?
Dealerships for their part want to micmic existing logic and functionality. For example if a customer doesn't have their car associated to their phone number, the dealer will transfer them to someone who can set it up. Or if a customer mentions they have a recall, the caller needs to make a service appointment. These kinds of rules and controls need to be visible and explainable.
We have internal tools for viewing phone calls and the path they take through our various systems. We can see what the AI model would predict a response to be and when / if control logic takes over. Using these tools we can often come up with an explanation.
Observation
In traditional programming, if you want to know what led to a specific outcome, you can debug it. You can go through each step of the logic and observe what happens as you change things. There's no way to do that in AI system unless you built in those capabilities.
The threat of regulations to AI technology become more real as countries race to stay up to speed. Any kind of regulation would imply a need for some form of explainability to be compliant.
I'm glad Dr. Lee mentioned the field of Explainable AI in her book The Worlds I See. Once I read it, I knew this described things I do at work: explain the choices our AI systems make. Now I've gotten to explain explainability and that's just fun to say.